データサイエンスをやっているときに、マシンパワーが発揮できていないことに気がつきました。

この原因を調べた所、PythonのGIL(Global Interpreter Lock)が原因であることが分かりました。
ライブラリと拡張 FAQ — Python 3.7.3 ドキュメント
用語集 — Python 3.7.3 ドキュメント
このGILを回避するためにマルチプロセス化をしました。以下のサイトが参考になりました。
Python高速化 【multiprocessing】【並列処理】 – Qiita
multiprocessing — プロセスベースの並列処理 — Python 3.7.3 ドキュメント
具体的にはmultiprocessingパッケージを利用してコードを書き直しました。
変更前のソース
import asyncio import tqdm import MeCab import numpy as np from multiprocessing import Pool tab_all = [] # 時間がかかる処理を含む関数 def handle(t): strs = mecab.parse(t).split('n') table = [s.split() for s in strs] table = [row[:4] for row in table if len(row) >= 4] if len(table) == 0: return tab = np.array(table) tab_all.append(tab[:,[0,3]].tolist()) if __name__ == '__main__': mecab = MeCab.Tagger("-Ochasen") f = open('jawiki_small.txt').readlines() text = [s.strip() for s in f] for t in text: handle(t)
変更した箇所
if __name__ == '__main__': mecab = MeCab.Tagger("-Ochasen") f = open('jawiki_small.txt').readlines() text = [s.strip() for s in f] with Pool(processes=8) as pool: pool.map(handle, text)
実行するとCPUがフルで利用されていることが分かります。(メモリが厳しいので増設したほうが良さそう…)

実行時間を比較したところ 1/3 程度まで削減できたことが分かります。
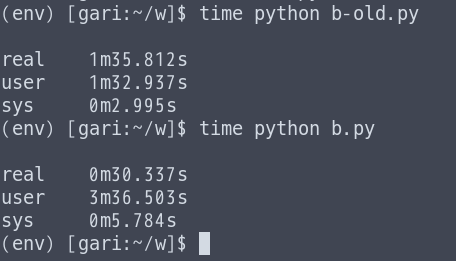
Pythonの裏側を理解することの大切さを学びました。
コメントを残す